Congress participation
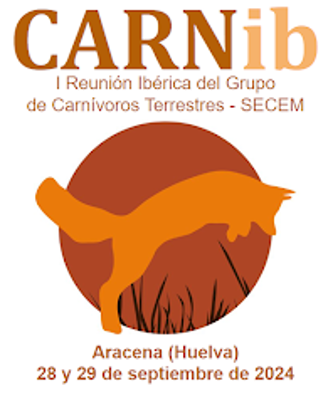
1st Iberian meeting of the Terrestrial Carnivores Group, SECEM – 28 and 29 September 2024 – Aracena, Spain
Dog invasions in protected areas. A study using camera traps, citizen science and artificial intelligence
Santiago Gutiérrez-Zapata, Simone Santoro, Manuel Emilio Gegúndez, Nuria Selva, Javier Calzada
Abstract
Domestic dogs (Canis familiaris) pose a serious threat to wildlife: they disturb it, prey on it, transmit diseases, and generally disrupt the ecological balance of their ecosystems. This study examines the presence of dogs in one of Europe’s most well-protected natural areas, Doñana National Park (Huelva, Spain), where the presence of dogs is strictly prohibited. We describe and assess the extent of the park’s dog infestation and investigate whether the presence of dogs is related to potential access routes. To this end, we searched for the presence of dogs in 5,200,000 photos collected by 60 camera traps randomly distributed throughout Doñana from October 2020 to January 2024. To locate the dogs, the images were pre-classified by a citizen science platform and an artificial intelligence system, and subsequently validated by experts. We found up to 33 different dogs within the protected natural area. Most of the time, the dogs roamed in packs of 2-5 individuals. Some dogs wandered through the park for up to 9 months over an area of 10 to 42 km². The probability of detecting dogs increased near one of the villages surrounding the park. We identified three types of dogs roaming in Doñana: dogs accompanying poachers, dogs owned by nearby villagers but allowed to roam freely, and stray dogs with no owner. We believe urgent measures must be taken to address the invasion of dogs in Doñana. In fact, dogs have been involved in the death of at least six adult female Iberian lynxes (Lynx pardinus) in Doñana. Regular monitoring of dog presence in protected natural areas is crucial to prevent invasions and their associated impacts
Integrating photo-trapping, artificial intelligence and citizen science to understand species coexistence
Santiago Gutiérrez-Zapata, Simone Santoro, Manuel Emilio Gegúndez-Arias, Nuria Selva, Javier Calzada
Abstract
According to the competitive exclusion principle, two ecologically similar species cannot coexist in the same space unless they develop strategies and adaptations that allow them to diverge or coexist within their ecological niche, either spatially, temporally, or trophically. Our study aimed to describe the daily activity patterns of the carnivore community (Iberian lynx, Lynx pardinus; red fox, Vulpes vulpes; Egyptian mongoose, Herpestes ichneumon; badger, Meles meles; and genet, Genetta genetta) and two of their potential prey species (rabbit, Oryctolagus cuniculus; and hare, Lepus granatensis) in Doñana National Park, as well as to evaluate the degree of overlap in these activity patterns between species.
Between January 2021 and January 2024, we deployed 38-60 camera traps in the non-floodable area of Doñana National Park. The image classification process was carried out through a citizen science project, a convolutional neural network, and subsequent verification by experts. In total, we obtained 3,307 independent detections at the species level.
We found predominantly nocturnal activity for all species, except for the Egyptian mongoose, which was entirely diurnal. Among the carnivores, we observed a high (Δ₁ > 75th percentile) to moderate (Δ₁ = 50th percentile < 75th percentile) temporal overlap, except when compared to the Egyptian mongoose, which occupied a different time niche. Regarding the overlap with prey, a greater temporal overlap was found between the rabbit and the predators than between the hare and the predators. Our results suggest that coexistence among the carnivore community in Doñana National Park may be facilitated by subtle differences in the species’ circadian rhythms, but other factors must also play a role. Furthermore, our findings demonstrate that integrating camera trapping with artificial intelligence and citizen science allows for the study of species coexistence mechanisms.”
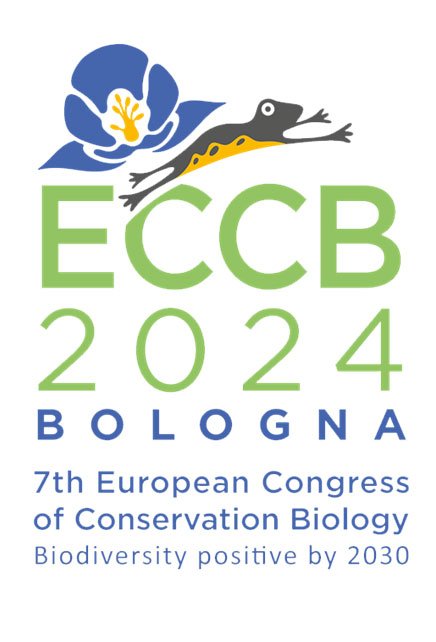
7th European Congress of Conservation Biology – “Biodiversity positive by 2030”19 June 2024 – Bologna, Italy
Classification performance in camera trap photos varies across citizen science, artificial intelligence, class types and environment
Abstract
1. Camera trapping, a popular non-invasive wildlife monitoring tool, produces numerous images. The overlooked variation in classification performance, which affects ecological inference, arises from using systems like Citizen Science (CS) and Convolutional Neural Networks (CNNs), class types, and camera/environment factors.
2. We evaluated a Citizen Science project and two CNN architectures on a dataset of 100,059 expert-classified images, identifying diurnal and nocturnal images across six classes: humans, cervids, leporids, wild boars, red foxes, and empty images.
3. Citizen Science showed high precision, while CNNs had a superior recall, particularly for leporids. Both struggled with nocturnal photos, especially in precisely identifying empty images, indicating possible under-detection at night. CNNs processed up to 1,150,000 images daily, far outpacing Citizen Science, which annotated only 25% of the same volume. Filtering out low-confidence classifications leads to an impractical increase in the manual workload, lowering the overall detection rate due to fewer images for reliable identification.
4. These findings highlight a key distinction: CNNs effectively maximize species detection with speed and high recall, whereas Citizen Science is better for minimizing classification errors. The study underscores the need to evaluate misclassification variations across systems, classes, and environmental factors for accurate ecological inference.